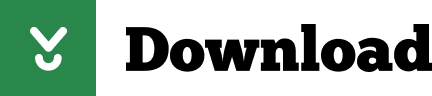
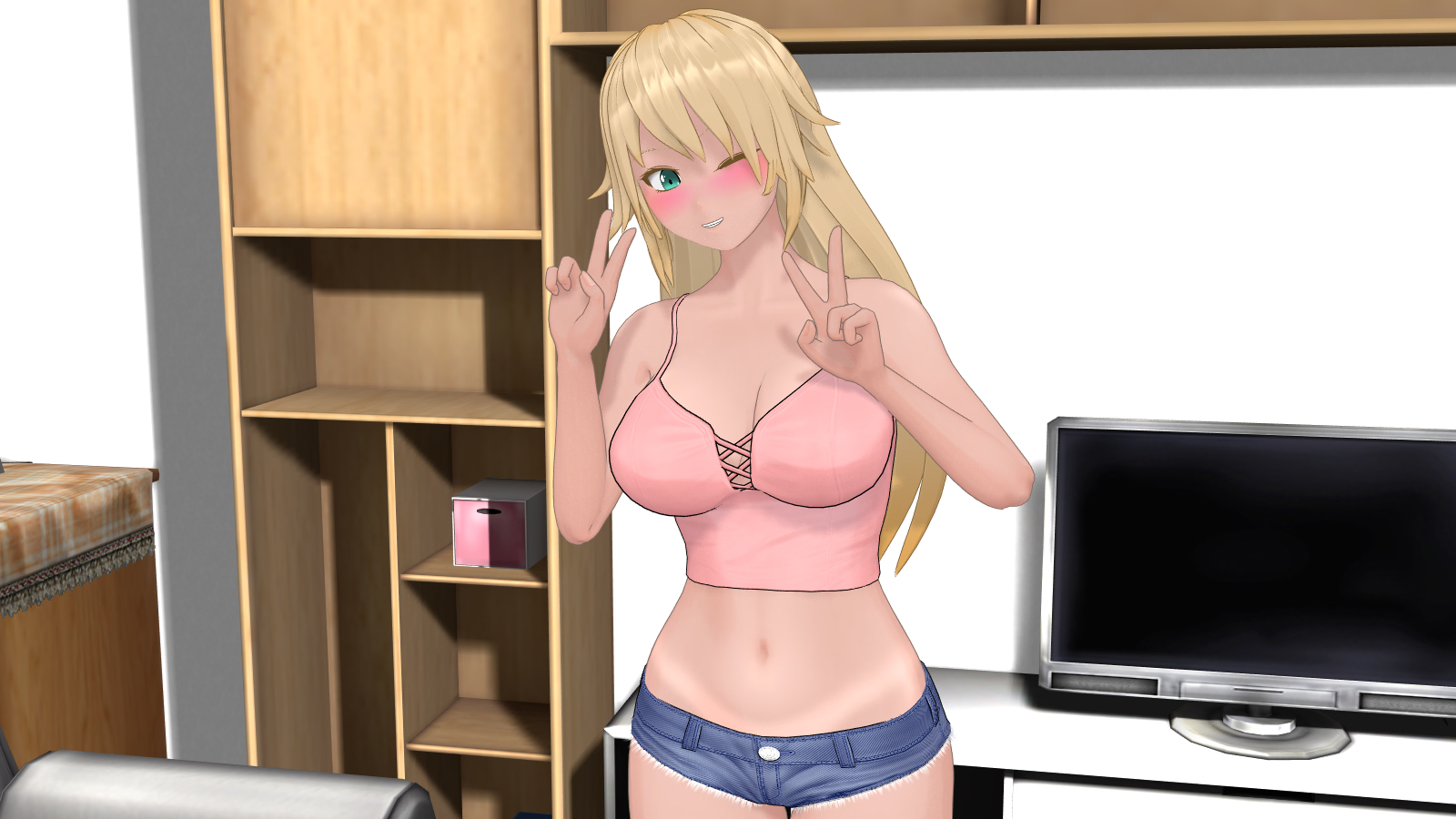
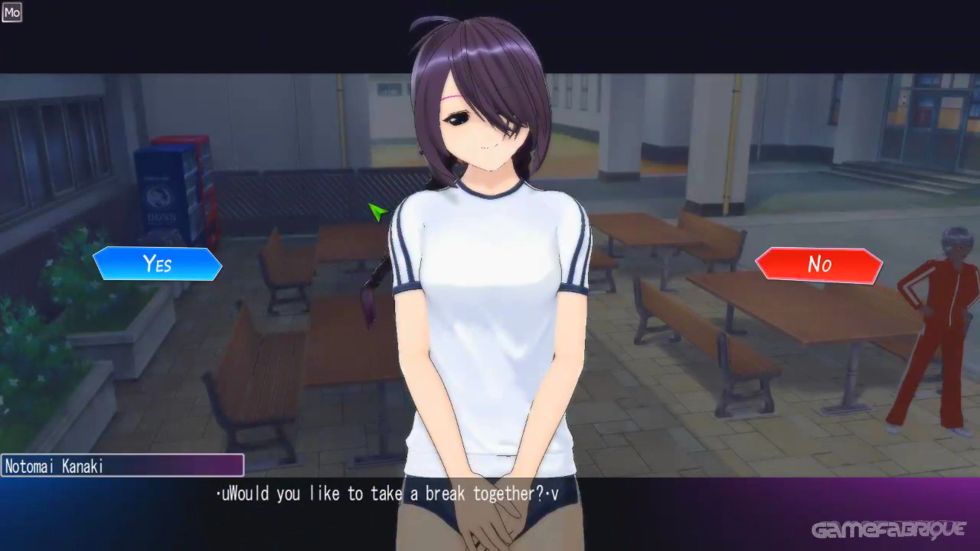
Each OnlineOpen article will be subject to a one-off fee of £1525 (equivalent to US$3000) to be met by or on behalf of the Author in advance of publication.
Artificial academy 2 save editor download#
free for all to view and download via the Wiley Online Library. OnlineOpen is a pay-to-publish service from Wiley-Blackwell that offers authors whose papers are accepted for publication the opportunity to pay up-front for their manuscript to become open access (i.e. Please still send on a completed Copyright Transfer Agreement from the link above. If you wish your paper to be OnlineOpen you are required to complete the payment form available from our website at.
Artificial academy 2 save editor license#
You are required to complete the standard Copyright Transfer Agreement, the author identified as the formal corresponding author for the paper will receive an email prompting them to login into Author Services where via the Wiley Author Licensing Service (WALS) they will be able to complete the license agreement on behalf of all authors on the paper. In accordance with accepted practice, the research findings reported in this paper must not appear in another publication before its publication in this Journal. The manuscript now becomes the copyright of the Journal. We look forward to your continued contributions to the Journal.

It is a pleasure to accept your manuscript entitled "Application of artificial intelligence and machine learning for prediction of oral cancer risk." in its current form for publication in the Journal of Oral Pathology and Medicine. The results demonstrate that the artificial neural network could perform well in estimating the probability of malignancy and improve the positive predictive value that could help to predict the individuals' risk of developing OC based on knowledge of their risk factors, systemic medical conditions, and clinic-pathological data. The accuracy of ANN for oral cancer prediction was 78.95% (95% CI, 54.43-931.95).Conclusion: Our results suggest that this machine-learning technique has the potential to help in oral cancer screening and diagnosis based on the datasets. Our analysis displayed that the average sensitivity and specificity of ANN for oral cancer prediction based on the 10-fold cross-validation analysis was 85.71% (95% confidence interval, 57.19-98.22) and 60.00% (95% CI, 14.66-94.73), respectively. Thirty-seven were female, and 36 were male, with a mean age of 63.09 years. Twenty-two (30.13%) were benign cases, and 51 (69.86%) were malignant cases. All records and observations were reviewed by Board-certified oral pathologist.Results: A total of 73 patients met the eligibility criteria. The dataset was randomly split into the training dataset 54 (75%) cases and testing dataset 19 (25%) cases. A total of 29 variables that were associated with the patients were used for developing the model. This study aimed to develop an artificial neural network model that helps to predict the individuals' risk of developing oral cancer based on data on risk factors, systematic medical condition, and clinic-pathological features.Methods: A popular data mining algorithm artificial neural network was used for developing the artificial intelligence-based prediction model. Background: Oral cancer requires early diagnosis and treatment to increase the chances of survival.
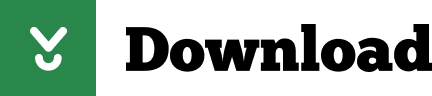